Balancing External vs. Internal Validity: An Application of Causal Forest in Finance
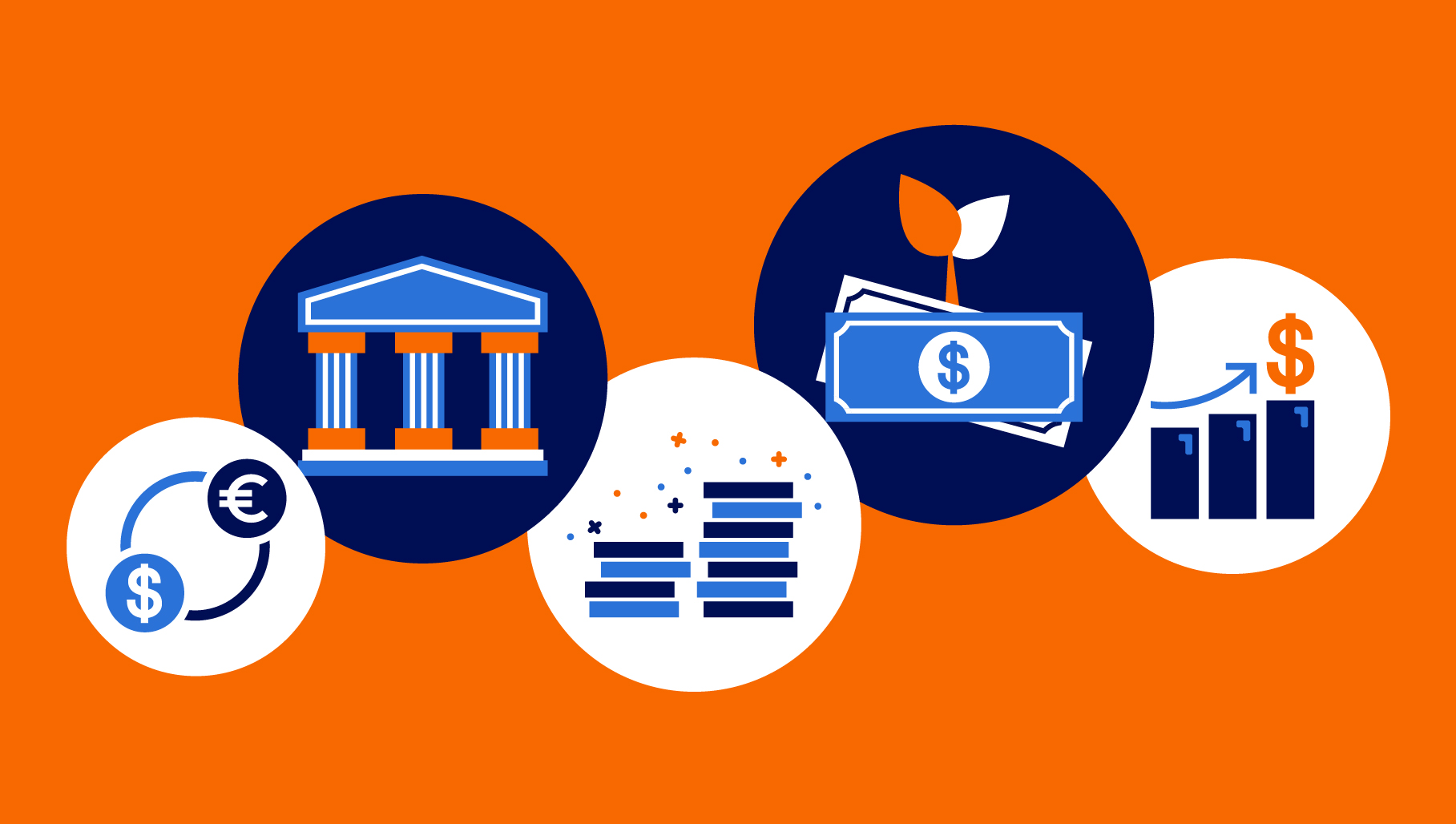
Author(s) Information:
Candace Jens, Whitman School of Management, Syracuse University
Huseyin Gulen, Mitch Daniels School of Business, Purdue University
T. Beau Page, Office of the Comptroller of the Currency
Journal (Year):
Management Science (2024)
Summary:
We can use causal forest, a new estimator with foundations in machine learning, to provide more broad and general answers to research questions in finance than traditional estimators.
Research Questions:
1. How does causal forest perform at measuring effects relative to more traditional estimators, including ordinary least squares (OLS)?
2. How does causal forest perform at measuring effects relative to research discontinuity design (RDD)?
3. Can we characterize settings in which causal forest performs better than either OLS or RDD (or both)?
4. Using causal forest, rather than OLS or RDD, do we recover a different effect of debt covenant violation, or technical default, on firm investment? Can we explain differences in the effects that each estimator recovers?
What We Know:
In observational data, RDD focuses on a small sample in which treatment is as good as random to recover a local average treatment effect (LATE) that is unbiased, or that has strong internal validity. However, because RDD focuses on a small sample, LATE is imprecise (i.e., high variance) and has weak external validity, particularly if treatment effects are heterogeneous. Intuitively, the higher the variance of underlying treatment effects, the less likely LATE is close to an average effect in any other subsample of data. In contrast, machine-learning-based causal forest recovers precise (i.e, low variance) observation-level effects that can be averaged across any subsample of interest to provide conditional average treatment effects (CATEs). CATE for observations within the bandwidth sample should approximate RDD LATE, and CATEs outside of the bandwidth, along with information that a forest recovers on drivers of treatment effect heterogeneity, demonstrate the extendability of bandwidth CATE and RDD LATE. While any RDD can be augmented with causal forest estimates, the benefits of causal forest are greatest when variation in treatment effects reduces the generalizability of RDD LATE. By combining imprecise but unbiased RDD estimates with causal forest's precise estimates with low (but probably not zero) bias, researchers can strike a better balance between bias and variance, or internal and external validity, than with RDD alone. In this paper, we identify settings in which causal forest estimates are relatively low-bias and can be used either instead of or alongside RDD to provide answers to research questions.
Novel Findings:
Our paper makes three contributions. First, we introduce into the finance literature causal forest, with a focus on the strengths of the estimator that drive its ability to recover low-bias and low-variance treatment effects. Second, we use Monte Carlo experiments in simulated data to provide the first empirical evidence of causal forest's effectiveness when treatment is endogenous. While causal forest is not an endogeneity panacea, we demonstrate the sources of bias to which causal forest estimates are robust and how to quantify potential bias in forest estimates, so that, in any setting, the benefits of causal forest's heterogeneous, extendable estimates can be weighed against the possibility of bias. Third, we use our Monte Carlos to inform our selection of a setting for an application of causal forest: the effect of loan covenant default on firm investment. Because of concerns about bias driven by differences between firms in and not in default, previous literature uses RDD to answer this question. Our application provides a road map for applied researchers interested in using causal forest alongside RDD in observational data to enhance inferences.
Full Citation:
Gulen, H., Jens, C., Page, T. B., 2024. Balancing external vs. internal validity: An application of causal forest in finance. Management Science, forthcoming.
Abstract:
Answering causal questions with generalizable results is challenging. Estimators requiring pseudo-randomization provide estimates with no bias (i.e., strong internal validity) but limited generalizability (i.e., weak external validity). Theoretically, causal forest, a non-parametric, machine-learning-based matching estimator, can provide low-to-no-bias, generalizable estimates even when treatment is endogenous. We empirically compare the performance of OLS, regression discontinuity design (RDD), and causal forest at recovering estimates in simulated observational panel data and show the robustness of causal forest estimates to many sources of bias. We re-visit a popular RDD setting, debt covenant default, to show how extendable, heterogeneous causal forest estimates can enhance inferences.