In the News and Trending: AI and Machine Learning: Can Substituting Human Decision Making with Machine Learning Negatively Impact Organizational Learning?
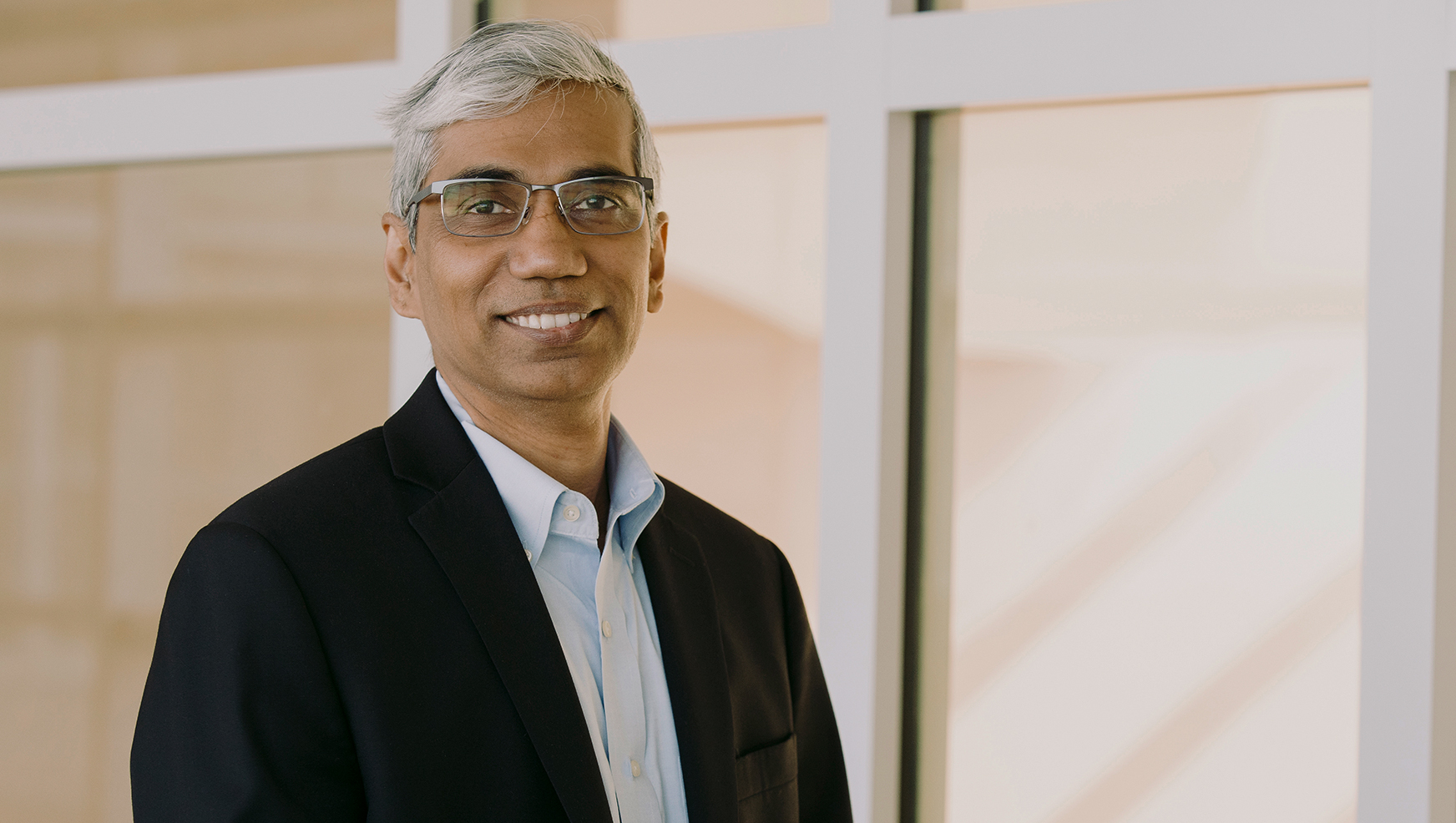
The perspectives of accomplished Whitman professors on timely issues impacting business. Stated wording and opinions are those of the author.
Natarajan Balasubramanian is a professor of management at the Whitman School. His research has been published in Management Science, Review of Economics & Statistics, and Strategic Management Journal. Balasubramanian aims to enhance our understanding of economic value creation by new and existing firms.
Artificial intelligence (AI) is increasingly all around us, as is the uncertainty of what aspects of AI are beneficial or detrimental. Is it making our jobs easier or rendering many of us obsolete? Will it impact the way we do business forever? Today, there are more questions than answers, but one thing is certain: AI is not going away.
One of my studies is on the organizational implications of machine learning (ML), a subset of AI, which uses large volumes of data to generate statistical models that are used to make decisions without human intervention. In “Substituting Human AI and Machine Learning: Can Substituting Human Decision Making with Machine Learning Negatively Impact Organizational Learning. “Decision Making with Machine Learning: Implications for Organizational Learning” with Yang Ye, Southwestern University of Finance and Economics, and Mingtao Xu, Tsinghua University (published in Academy of Management), we explore how replacing human decision making with ML can alter the richness and diversity of organizational learning.
Let’s say, for example, a business receives 100 applications for a job opening. How long would it take someone to examine each one and choose the five most qualified candidates? Hours? Days? In contrast, ML can complete the process in a few seconds using statistical models trained on historical data on similar decisions. Clearly, it’s more efficient, but what about the risk that an excellent candidate is passed over simply because they do not fit the statistical model embedded in the ML algorithm?
My research proposes that substituting human decision making with ML risks impoverishing learning in an organization by decreasing both the diversity inherent in organizational routines and the extent of casual, contextual and general knowledge associated with these routines. By downplaying individual experiences and relying on narrow statistical criteria, ML can amplify the risk of overly focusing on the here and the now, a phenomenon also known as learning myopia.
ML makes decisions, in the simplest terms, using models that ask, “Given x, what is the most likely y?” It answers this question using models that are best from historical and statistical perspectives. The risk then is that a diversity of views is eliminated and squeezed into a single or narrow set of perspectives chosen to fit those models. In this way, ML risks becoming detrimental to organizational learning. In contrast, human decision making allows for a process to improve with experience, as people can incorporate what they learn, share varied individual world views and experiences, and consider different paths through trial and error.
Certainly, and especially with the availability of recent generative AI tools that we did not consider in our research, the argument can be made that human experiences and knowledge can be better internalized in algorithms and that ML will continue to become more sophisticated in its decision making. However, the fact remains that notwithstanding their cognitive limitations and biases, in many contexts, humans are still more capable of recognizing, understanding and correcting mistakes, and of considering a wider array of options, including the elimination of inappropriate ones.
A key implication of our study is that organizations have to cautiously evaluate decisions to substitute human decision making with ML, judiciously balancing process efficiency with the need for diversity of organizational learning. Carefully considering where the diversity of human thinking may be beneficial and taking steps to retain and nurture such diversity will, we think, help organizations mitigate the risk of learning myopia.
Additional AI-Related Research That Whitman Faculty Members Are Exploring:
Using Store AI to Enhance Consumer Purchase Decisions by Promoting Consumers Sense of Autonomy
By Associate Professor of Marketing Guiyang Xiong
Many retailers have started AI systems to help consumers choose the best fit products, streamlining and improving the purchasing decision-making process. This study addresses how consumers make purchase decisions with AI that models consumers’ personal preferences and needs but do not necessarily align with the consumers own preferences.
Adversarial Machine Learning Attacks on Financial Reporting By Professor of Management Information Systems
By Michel Benaroch, Professor of Accounting Johann Comprix and Edward Raff ’24 MBA
This research demonstrates a new application area that has motivated use of ML by attackers and realistic threat models: financial reporting fraud. The application shows why and how bad actors, primarily distressed firms, have the incentive to manipulate financial reports to hide their distress and derive personal gains in the form of millions of dollars and access to many publicly disclosed and used financial modeling frameworks.
Reproducibility and Scientific Rigor of Machine Learning and Business Relevance
By Professor of Management Information Systems Michel Benaroch and Edward Raff ’24 MBA
This ongoing research examines how scientific algorithms may produce different results through various, though seemingly equivalent, implementations, which has caused an increased concern in the AI and ML communities regarding reproducibility.
How Does Worker Mobility Affect Business Adoption of a New Technology? The Case of Machine Learning
By Professor of Management Natarajan Balasubramanian, Ruyu Chen, Stanford Institute for Human-Centered Artificial Intelligence, and Chris Forman, Cornell University (forthcoming at the Strategic Management Journal)
This research looks at how the adoption of ML by businesses varies with the likelihood of outward mobility of workers. When a technology is new, such as ML was until recently, only a few workers have the relevant technical skills and on-the-job experience, which can create sizable costs to businesses if those employees leave.